The Intersection of Hardware and Software in Edge AI
- shugran sabbahin
- Oct 27, 2024
- 6 min read
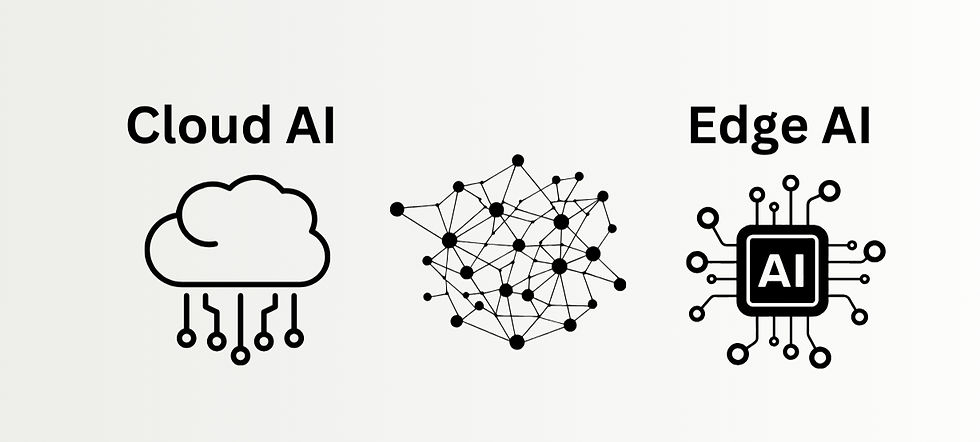
Artificial intelligence has been at the forefront of technological advancement for the last few decades. However, there is a growing shift toward Edge AI, which promises to enhance real-time decision-making and data processing directly on devices. The concept of Edge AI focuses on moving intelligence away from centralized data centers and bringing it to the “edge” of the network—where data is generated. This shift has profound implications for industries ranging from healthcare and autonomous driving to industrial IoT and smart cities.
In this blog, we will take a deep dive into Edge AI from a technical perspective, exploring its hardware and software components, understanding its use cases, and addressing the engineering challenges that come with deploying AI on the edge.
1. What is Edge AI?
Edge AI refers to the deployment and execution of artificial intelligence (AI) algorithms locally on devices that are located at the “edge” of the network. These edge devices include things like sensors, cameras, smartphones, and industrial equipment. In an Edge AI system, data processing, analytics, and AI model inference are done on the local hardware, instead of sending data to a centralized cloud server.
In traditional AI architectures, data is typically transmitted to large, cloud-based data centers where intensive computations occur, and the results are sent back to the devices. Edge AI eliminates this round-trip, enabling devices to make autonomous decisions in real-time. This characteristic makes Edge AI highly suitable for applications that require low latency, such as autonomous vehicles, industrial robotics, and healthcare diagnostics.
2. Why Edge AI? Benefits Over Traditional AI
As engineers, we're well aware that moving computations to the cloud has served us well for years. However, with the rise of IoT devices, sensors, and autonomous systems, it’s becoming increasingly necessary to process data at the point of collection. Here are some compelling reasons why Edge AI is gaining prominence:
Low Latency: In applications such as autonomous driving or industrial robotics, milliseconds matter. Sending data to the cloud and waiting for a response introduces delay. With Edge AI, processing happens on the device itself, reducing the response time and allowing for real-time decision-making.
Data Privacy and Security: With increasing concerns around data privacy, keeping data local is often a better solution. For example, in healthcare, patient data can be processed directly on a wearable device without being sent to external servers, reducing the risk of unauthorized access.
Bandwidth Optimization: As the number of IoT devices grows, the amount of data being transmitted to the cloud could overwhelm networks. Edge AI reduces the burden on the network by processing the majority of data locally, transmitting only what's necessary.
Offline Functionality: Many critical applications need to continue working even without a constant internet connection. Edge AI allows devices to function autonomously, even when they are offline or in areas with limited connectivity.
Scalability: Distributed AI at the edge allows organizations to scale their solutions more effectively, as the cloud is no longer a bottleneck.
3. Key Hardware Components of Edge AI
The success of Edge AI hinges on the capability of the hardware. Unlike cloud-based systems where there are virtually unlimited resources, edge devices must balance power consumption, processing power, and physical size constraints. Here’s a breakdown of key hardware components in an Edge AI system:
Microcontrollers (MCUs)
At the heart of many IoT and embedded systems are Microcontroller Units (MCUs). These are low-power processors designed for simple tasks such as controlling sensors, motors, or communications. They are not traditionally associated with AI processing, but with advancements in tinyML, it is now possible to run lightweight AI models directly on MCUs.
MCUs are favored in applications like wearable devices or low-power industrial sensors, where energy efficiency is critical. The trade-off, however, is that the models must be highly optimized, and more computationally intensive tasks may require additional hardware.
Graphics Processing Units (GPUs)
GPUs have been a mainstay for AI processing due to their ability to handle large-scale parallel computations, particularly in training deep learning models. When deployed on edge devices, GPUs are primarily used for inference (i.e., making predictions from pre-trained models).
In Edge AI systems, GPUs are ideal for applications that require processing large amounts of visual or sensory data, such as drones, autonomous vehicles, or security cameras. The challenge lies in balancing the GPU's power consumption with the need for performance, especially in battery-powered edge devices.
Field-Programmable Gate Arrays (FPGAs)
One of the more flexible hardware options for Edge AI is Field-Programmable Gate Arrays (FPGAs). These chips can be reprogrammed after manufacturing to meet the specific needs of different AI tasks. FPGAs strike a balance between performance and energy efficiency, making them a versatile choice for edge applications.
One key advantage of FPGAs is that they can be tailored for specific AI models or tasks, allowing engineers to optimize the hardware for the model's exact needs. This flexibility is particularly valuable in industries where AI models may change or evolve over time.
AI-Optimized ASICs
For applications requiring maximum efficiency, Application-Specific Integrated Circuits (ASICs) are a common choice. These are custom-built chips optimized for specific AI tasks. Unlike FPGAs, ASICs cannot be reprogrammed after manufacturing, but they offer superior performance and energy efficiency for AI inference tasks.
Companies like Google (with their TPU chips) and NVIDIA have developed AI-optimized ASICs to meet the demands of edge computing. These chips are designed to handle AI workloads such as object detection, speech recognition, or natural language processing with minimal power consumption.
4. Software Challenges and Considerations in Edge AI
While hardware plays a crucial role in Edge AI, the software side of the equation is equally important. AI models that run efficiently on cloud infrastructure often need to be adapted or compressed to function well on edge devices. Below are some of the software challenges and considerations that engineers must take into account when developing for Edge AI:
AI Model Optimization Techniques
Running AI models on edge devices often requires substantial model optimization to fit within the constraints of the hardware. Some common optimization techniques include:
Quantization: This process involves reducing the precision of the model's parameters (e.g., from 32-bit floats to 8-bit integers) to decrease memory usage and computation time.
Pruning: Pruning reduces the size of the neural network by removing unnecessary neurons or connections that have little impact on the model's performance.
Knowledge Distillation: In this technique, a smaller, more efficient model is trained to replicate the behavior of a larger, more complex model. The smaller model is then deployed on the edge device.
Model Partitioning: In some cases, parts of the model can be run on the edge device, while the rest is executed in the cloud. This hybrid approach can balance performance and resource constraints.
Each of these techniques comes with trade-offs in terms of accuracy, performance, and complexity, and the best solution depends on the specific use case.
Real-time Operating Systems (RTOS) and Middleware
Edge devices often need specialized operating systems and middleware to ensure that AI tasks run smoothly alongside other processes. Real-time Operating Systems (RTOS) are designed to manage tasks with strict timing requirements, making them ideal for time-sensitive applications like autonomous vehicles or medical devices.
Middleware plays an important role in managing communications between the hardware, the AI models, and the cloud. In many cases, engineers need to develop custom middleware to ensure efficient data flow and resource management in Edge AI applications.
Frameworks for Edge AI
Several frameworks have been developed to support the deployment of AI models on edge devices. These frameworks are optimized for different hardware platforms and come with tools for model optimization, quantization, and deployment. Some popular Edge AI frameworks include:
TensorFlow Lite: A lightweight version of TensorFlow designed for mobile and edge devices. It supports quantization and pruning, making it well-suited for running AI models on resource-constrained devices.
PyTorch Mobile: PyTorch’s mobile version allows developers to deploy AI models on both Android and iOS devices. It also includes tools for model optimization and quantization.
ONNX Runtime: An open-source framework that supports a variety of hardware platforms, including CPUs, GPUs, and FPGAs. ONNX models can be converted from different AI frameworks, providing flexibility in deployment.
Engineers must choose the right framework based on their hardware platform, AI model, and the specific needs of the application.
Conclusion
The convergence of hardware and software in Edge AI presents an exciting frontier for engineers and developers. By moving intelligence closer to where data is generated, Edge AI promises to enable real-time decision-making, reduce latency, and improve data privacy, all while reducing the burden on centralized cloud infrastructure.
For engineers working on Edge AI solutions, the key challenges lie in optimizing both hardware and software to fit within the constraints of edge devices, ensuring real-time performance, and maintaining security. As technologies like 5G, custom ASICs, and advanced AI models evolve, Edge AI will continue to drive innovation across industries, reshaping how we interact with and leverage intelligent systems.
Comments