Microcontroller Units (MCUs) in Edge AI: Empowering Intelligent Solutions at the Edge
- shugran sabbahin
- Oct 27, 2024
- 4 min read
Introduction
In an increasingly interconnected world, the role of artificial intelligence is shifting from centralized data centers to the edge of the network. This transformation—often called "Edge AI"—is reshaping industries by enabling real-time decision-making where data is generated. At the heart of this revolution are microcontroller units (MCUs), compact and energy-efficient computing platforms that are becoming indispensable for intelligent edge devices. In this blog, we will explore the role of MCUs in Edge AI, delve into the technical aspects that make them suitable for AI applications, and discuss some innovative use cases and challenges.
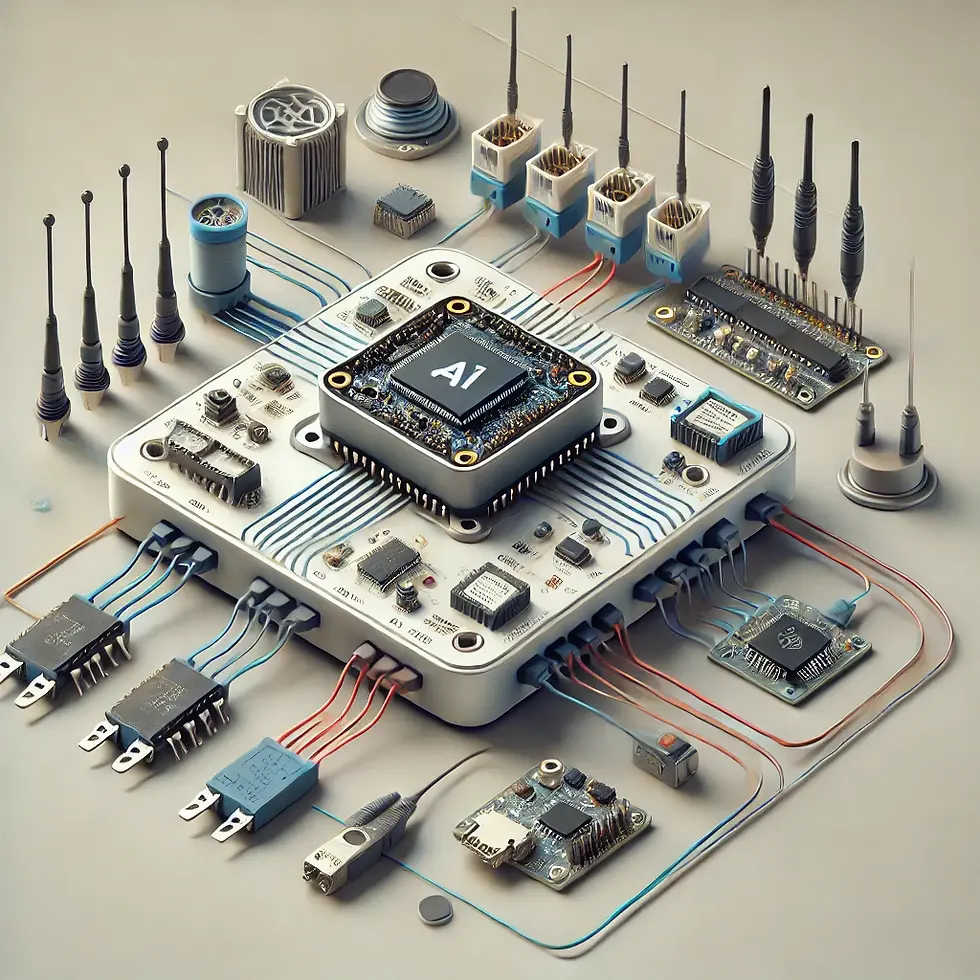
The Evolution of MCUs in Edge AI
MCUs have been around for decades, serving as the core of countless embedded systems, from household appliances to automotive controllers. Traditionally, MCUs were used to execute simple tasks like controlling motors, reading sensors, and managing communication protocols. However, with advances in semiconductor technology, MCUs have become powerful enough to support more complex computations, such as running AI models.
Modern MCUs can execute machine learning (ML) inference tasks at the edge, thanks to improvements in clock speeds, processing power, and specialized hardware like digital signal processors (DSPs) and machine learning accelerators. Unlike high-power CPUs and GPUs, MCUs are known for their energy efficiency, making them ideal for edge applications that require long battery life or operate in remote areas without stable power supply.
Comparison Table: Traditional MCUs vs Modern MCUs vs Edge Processors

Technical Foundations of MCUs for Edge AI
Low Power Consumption
MCUs are designed to operate with minimal power, often in the milliwatt range. This makes them highly suitable for battery-powered IoT devices and wearables. Energy efficiency is critical for deploying AI at the edge, where the goal is to minimize data transfer to the cloud while still executing complex tasks. By integrating sleep modes and efficient power management techniques, MCUs enable real-time AI inference with minimal energy use.
Integrated Peripherals and Connectivity
MCUs typically come with integrated peripherals such as Analog-to-Digital Converters (ADCs), Pulse Width Modulation (PWM) units, and multiple communication interfaces (e.g., I2C, SPI, UART). These peripherals allow MCUs to interface seamlessly with sensors, cameras, and actuators. Connectivity options, such as Bluetooth, Wi-Fi, and LoRa, also enable data sharing between edge devices, enhancing their overall capabilities.
Specialized Hardware for AI Acceleration
The latest generation of MCUs includes specialized hardware that can accelerate AI computations. Examples include DSP cores and even dedicated neural processing units (NPUs) designed to handle the specific mathematical operations involved in neural networks, such as convolutions and activation functions. These accelerators allow MCUs to run deep learning inference models, such as convolutional neural networks (CNNs), with improved efficiency and speed.
AI Workloads on MCUs: The Edge Use Cases
The capabilities of MCUs have enabled new and innovative AI use cases across various industries. Let’s look at some examples:
Smart Agriculture
In smart agriculture, MCUs are used to process data from soil sensors, weather stations, and cameras. By leveraging AI models trained to detect crop diseases or assess soil conditions, MCUs can make real-time decisions that help optimize irrigation, reduce pesticide use, and ultimately increase crop yield.
Predictive Maintenance
Predictive maintenance in industrial settings requires continuous monitoring of machine parameters, such as vibration and temperature. MCUs with built-in AI inference capabilities can process this sensor data locally, detecting anomalies and predicting equipment failures before they happen. This results in lower downtime and reduced maintenance costs.
Human Activity Recognition
Wearable devices, such as fitness trackers, benefit from MCU-powered AI for human activity recognition. These MCUs can process data from accelerometers and gyroscopes to classify activities like walking, running, or sitting in real-time, all while maintaining low power consumption, which is crucial for wearables.
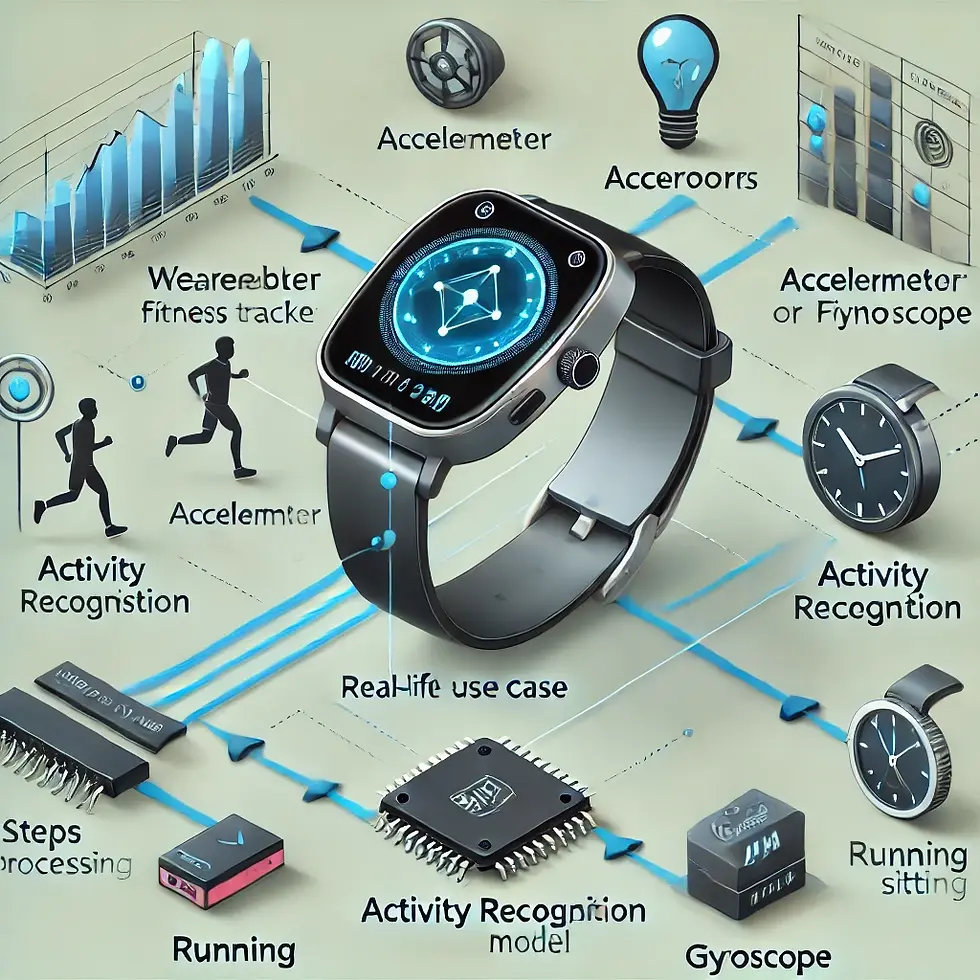
Challenges and Innovations
While MCUs are crucial in driving Edge AI, they come with their own set of challenges:
Limited Memory and Processing Power: The constrained memory (typically in kilobytes) and computational capabilities of MCUs pose challenges when deploying larger neural networks. To address this, techniques such as model quantization, pruning, and knowledge distillation are often used to shrink the size of AI models to fit within MCU constraints.
Real-Time Requirements: Edge AI applications often require low latency, and real-time response is critical for scenarios like autonomous navigation or safety monitoring. MCU-based systems must be carefully designed to meet timing requirements, often by leveraging real-time operating systems (RTOS).
Power Management: Although MCUs are power-efficient by design, managing power intelligently remains a challenge, especially in environments where continuous data processing is required. Innovations in dynamic voltage and frequency scaling (DVFS) are helping address this by allowing the MCU to adjust its power profile depending on workload demands.
The Future of MCUs in Edge AI
The future of MCUs in Edge AI is promising, thanks to ongoing innovations in both hardware and software. We are seeing the emergence of AI frameworks tailored for MCUs, such as TensorFlow Lite for Microcontrollers and Edge Impulse, which provide the necessary tools to deploy neural networks on these compact devices easily.
Moreover, advancements in silicon technology will continue to push the boundaries of what’s possible. As new MCUs incorporate more sophisticated AI accelerators, we can expect to see even more complex AI models running at the edge, handling tasks that previously required cloud computing power.
The ability of MCUs to bring intelligence to low-power, resource-constrained environments will continue to drive growth in sectors like healthcare, smart cities, and industrial automation. With MCUs at the heart of Edge AI, we are stepping into a world where every device—no matter how small—has the potential to be intelligent, responsive, and impactful.
Conclusion
MCUs have transformed from simple control units to powerful enablers of artificial intelligence at the edge. They are at the forefront of a technological movement that is bringing real-time decision-making capabilities to the point where data is generated. By enabling energy-efficient, responsive AI solutions, MCUs are helping to create a more connected and intelligent world. As we continue to innovate in AI, semiconductors, and embedded systems, the role of MCUs in the edge landscape will only grow, solidifying their place in the next generation of intelligent applications.
Call to Action
If you're curious about integrating MCUs into your next Edge AI project, explore our detailed guides and resources on optimizing AI models for embedded systems. Join our growing community of engineers pushing the boundaries of what's possible at the edge.
Comments